A team of researchers in Boston is poised to make a significant breakthrough in lung cancer screening. Their Revolutionary technology is an Artificial Intelligence (AI) system named Sybil that can detect early indications of the disease several years before it is detectable on a doctor’s CT scan. Developed jointly by scientists at the Mass General Cancer Center and Massachusetts Institute of Technology, the new AI tool boasts impressive results, with one study showing it accurately predicts an individual’s likelihood of developing lung cancer in the following year between 86% to 94% of the time.
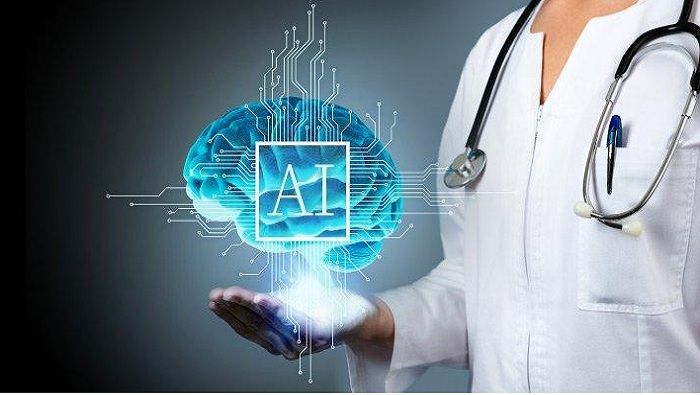
What is Sybil for Lung Cancer?
Dr. Lecia Sequist, the program director of the Cancer Early Detection and Diagnostics Clinic at Massachusetts General Hospital, emphasized the limitations of human observation by stating, “The naked eye cannot see everything.” She further explained that the AI tool, Sybil, can analyze CT scans in a distinct way compared to human radiologists.
The experts are hailing Sybil as a significant breakthrough in the early detection of lung cancer, which is the third most prevalent cancer in the United States, according to the CDC. The disease is also the leading cause of cancer-related deaths in the US, with the American Cancer Society estimating that more than 238,000 new cases and over 127,000 deaths from lung cancer are expected this year.
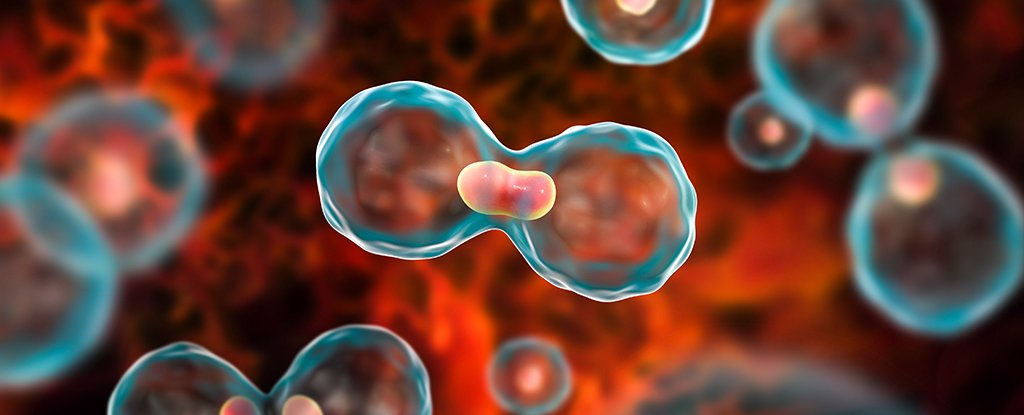
How does Sybil predict Cancer?
The researchers employed deep learning to create an AI model using data from 15,000 users. They trained and constructed their model using 35,001 low-dosage CT images, while 6,282 images were reserved for testing. To train the model, two thoracic radiologists identified worrisome lesions on scans of patients who developed cancer within a year of the scan. Sybil achieved an accuracy rate of 92% for detecting lung cancer across all test data sets after one year, 86% after two years, and a C-index likelihood of 75% after six years, utilizing only low-dose CT scans.
how accurately did Sybil identify lung cancer cases?
The researchers discovered that Sybil’s performance remained consistent across gender, age, and smoking history. They proceeded to assess Sybil’s capabilities using data from both Massachusetts General Hospital (MGH) in Boston, Massachusetts, and Chang Gung Memorial Hospital (CGMH) in Taiwan. Notably, CGMH patients were not required to have a smoking history for a low-dose CT scan, unlike the main and MGH datasets.
Based on the MGH dataset, Sybil correctly identified 86% of lung cancer cases or healthy lungs within a year, while the CGMH dataset, accurately identified 94% of patients. After six years, Sybil’s predictions were correct for 81% of lung tumors or healthy lungs in the MGH cohort and 80% in the CGHM cohort. The researchers assert that Sybil can also predict typical clinical risk factors such as smoking based on the scans analyzed.
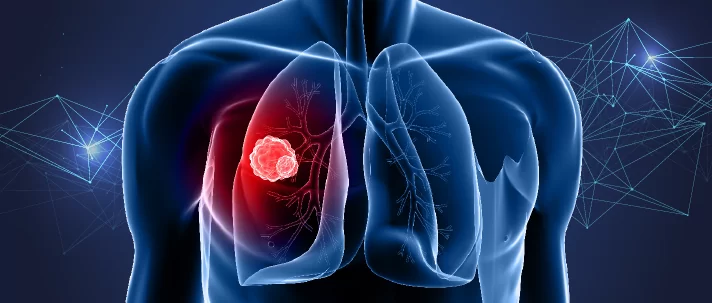
Limitations
The researchers acknowledged certain limitations in their model. They specifically pointed out that a significant proportion (92%) of the training data for Sybil was derived from White patients, implying that the model’s accuracy may not be generalizable to other racial groups. Additionally, they observed that the training data comprised CT scans obtained between 2002 and 2004, raising the possibility that Sybil’s predictive ability could be compromised by advances in CT technology since that time.
Conclusion
The ability of Sybil to predict lung cancer risk in non-smokers is uncertain due to the absence of specific smoking data from CGMH patients. The researchers anticipate that Sybil can potentially decrease the need for follow-up scans or biopsies in low-risk patients. Additionally, they plan to conduct a prospective clinical trial to assess Sybil’s effectiveness in the real world and its complementarity with radiologists’ tasks.
The researchers suggest that Sybil could operate in the background of radiology reading stations and forecast lung cancer risk using only low-dose CT scans without radiologists’ presence to annotate regions of interest or demographic and clinical data. The researchers have also publicly released the code.
read our more blogs on CEFinsights